The objective of subproject C02 is to develop methods that enable the reliable detection of structural changes in offshore megastructures during operation in order to minimize costly maintenance work.
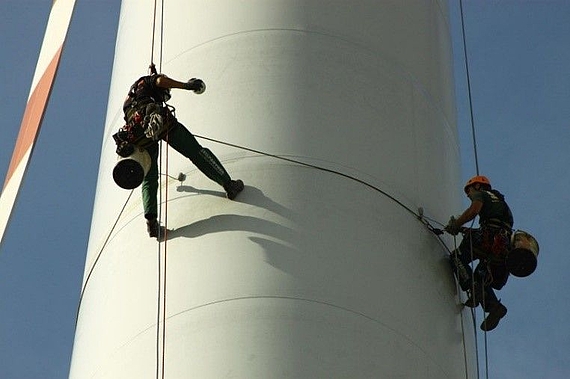
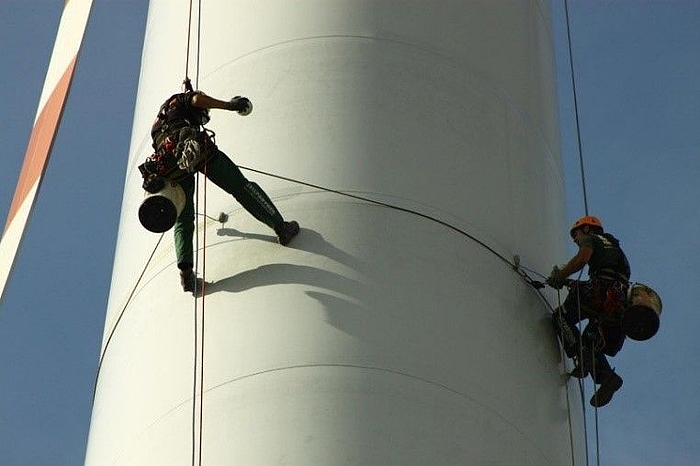
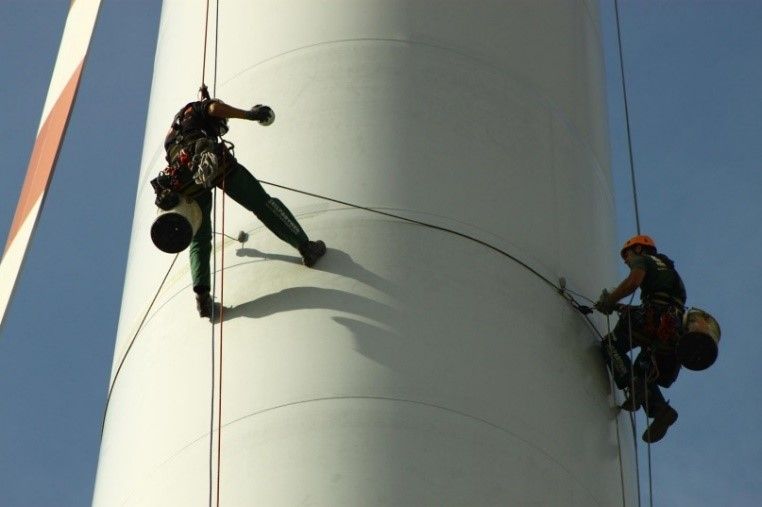
Structural changes can be, for example, undesired system changes (ice on the rotor blade) or potential damage (cracks). Here, the use of physically based finite element models is deliberately avoided. Instead, output only methods of system identification are used, for which only the measured response variables (accelerations, strains) and measurements of the environmental and operational conditions (temperature, rotor speed, wind speed, etc.) are considered.
The main challenges are that damage, or other critical system changes, cannot be measured directly, but can only be determined or estimated indirectly using damage-sensitive features. However, for a complex system such as an offshore wind turbine (OWEA), the number of feasible measurement points is small compared to the number of degrees of freedom of the structure. Thus, there is an information deficit. In addition, the structural dynamic behavior strongly depends on the environmental and operational conditions (EOCs). For example, interactions with aerodynamic forces occur at the rotor, which can lead to nonlinear vibration states. Therefore, classical methods of system identification, which assume linearity and time invariance of the metrologically observed system, cannot be readily applied. Moreover, a system change due to changing EOCs can easily be mistaken for damage.
On the other hand, in practical structural health monitoring (SHM), methods are urgently needed that allow rapid intervention in case of possible damage, but avoid unnecessary maintenance assignments due to false detections. Such maintenance operations are to be minimized as far as possible, especially in the offshore sector, due to the high accruing costs. The working hypothesis of the subproject (SP) is that a suitable combination of operational modal analysis (OMA) methods with physically interpretable state parameters and mathematical models from artificial neural networks (such as in Figure 1) can provide a suitable basis for structural monitoring of an offshore megastructure during operation.
The main innovation of the SP is the combination of robust algorithms based on white and black box models in a multivariate grey box SHM approach. Figure 2 shows a possible structure of a SHM- framework.
This concept will be tested and validated gradually on databases of structures of different complexity. These structures range from a 9m high lattice tower standing on an open field, which can be reversibly damaged (see Figure 3), up to a real 3.4MW onshore wind turbine with a rotor diameter of 104m and a hub height of 125m (see Figure 4).
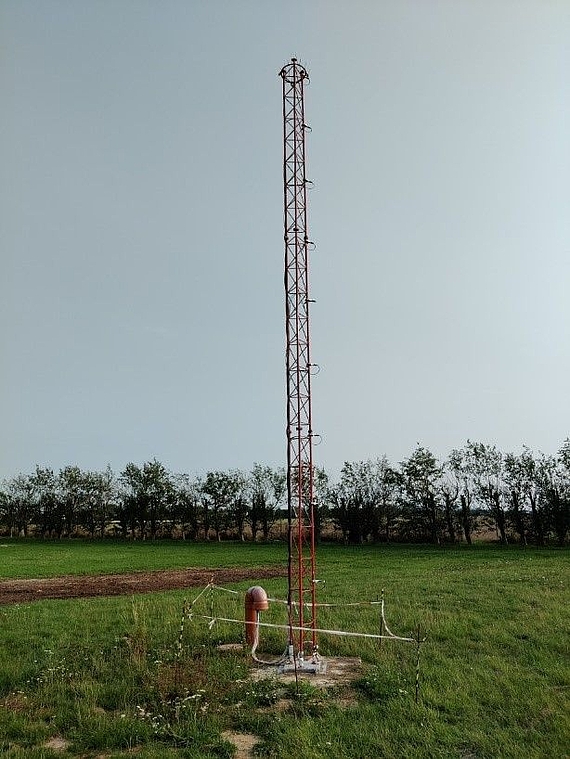
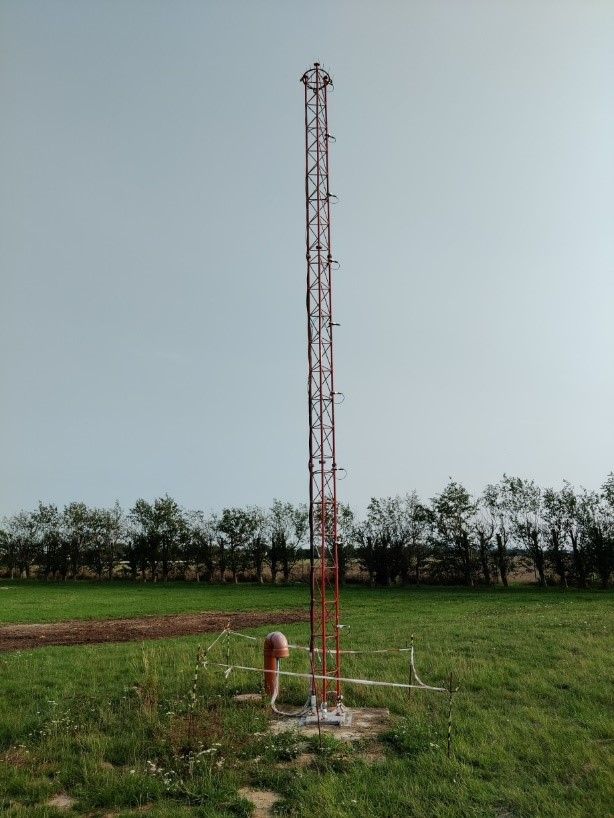
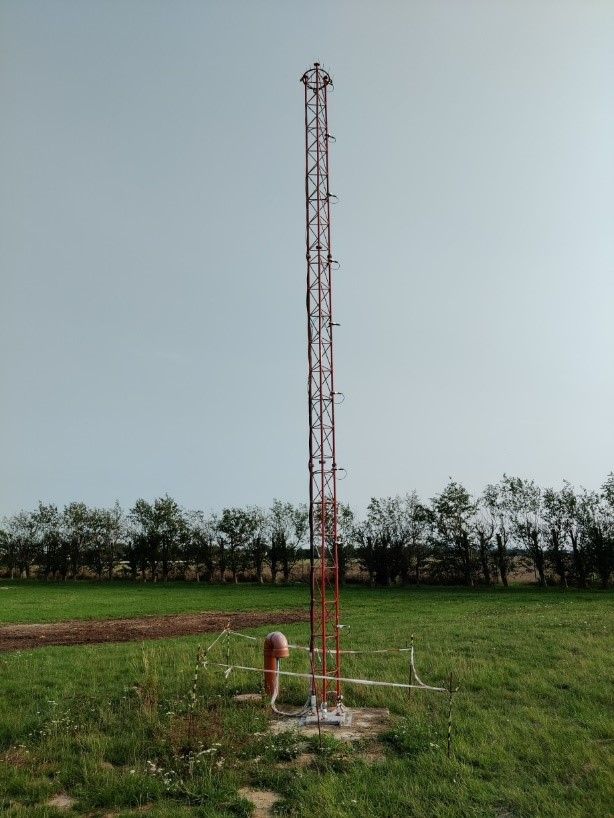
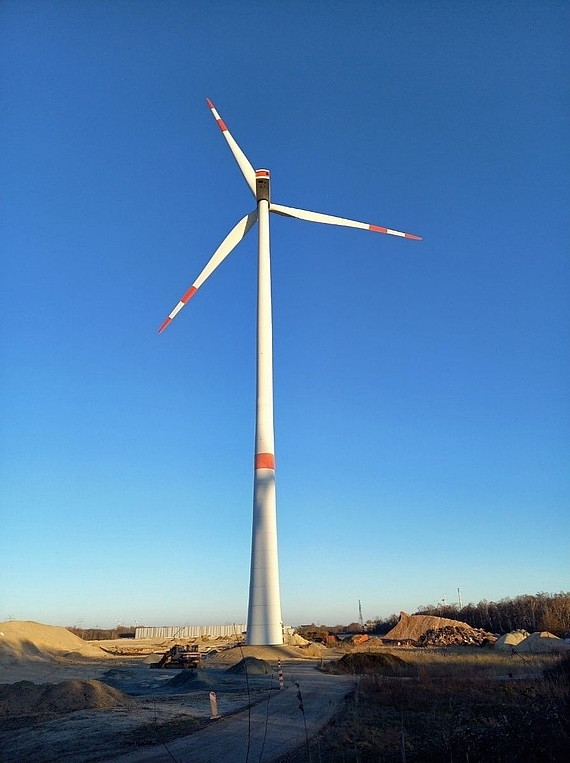
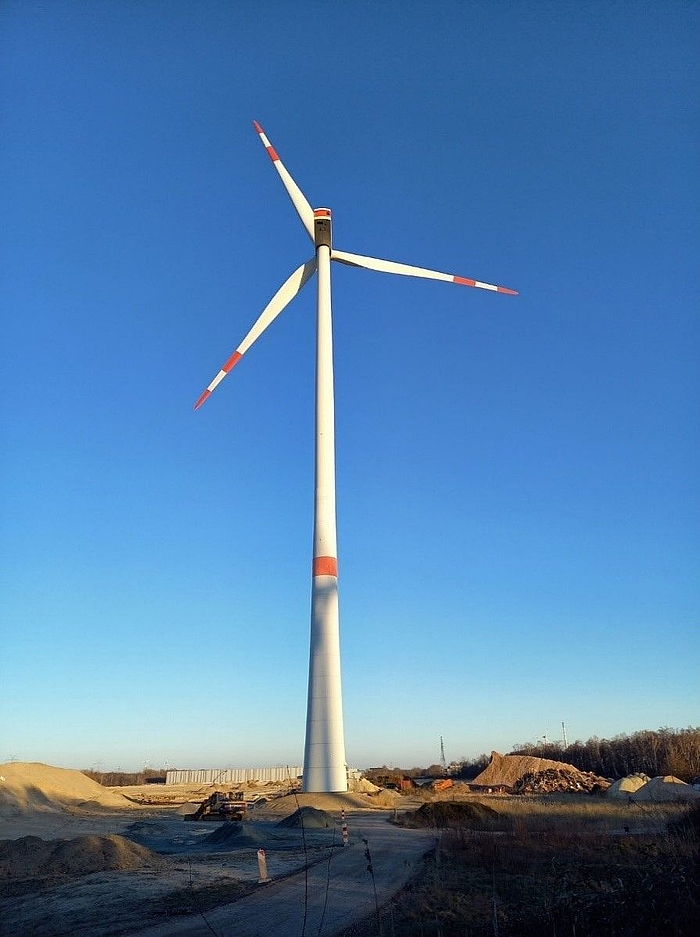
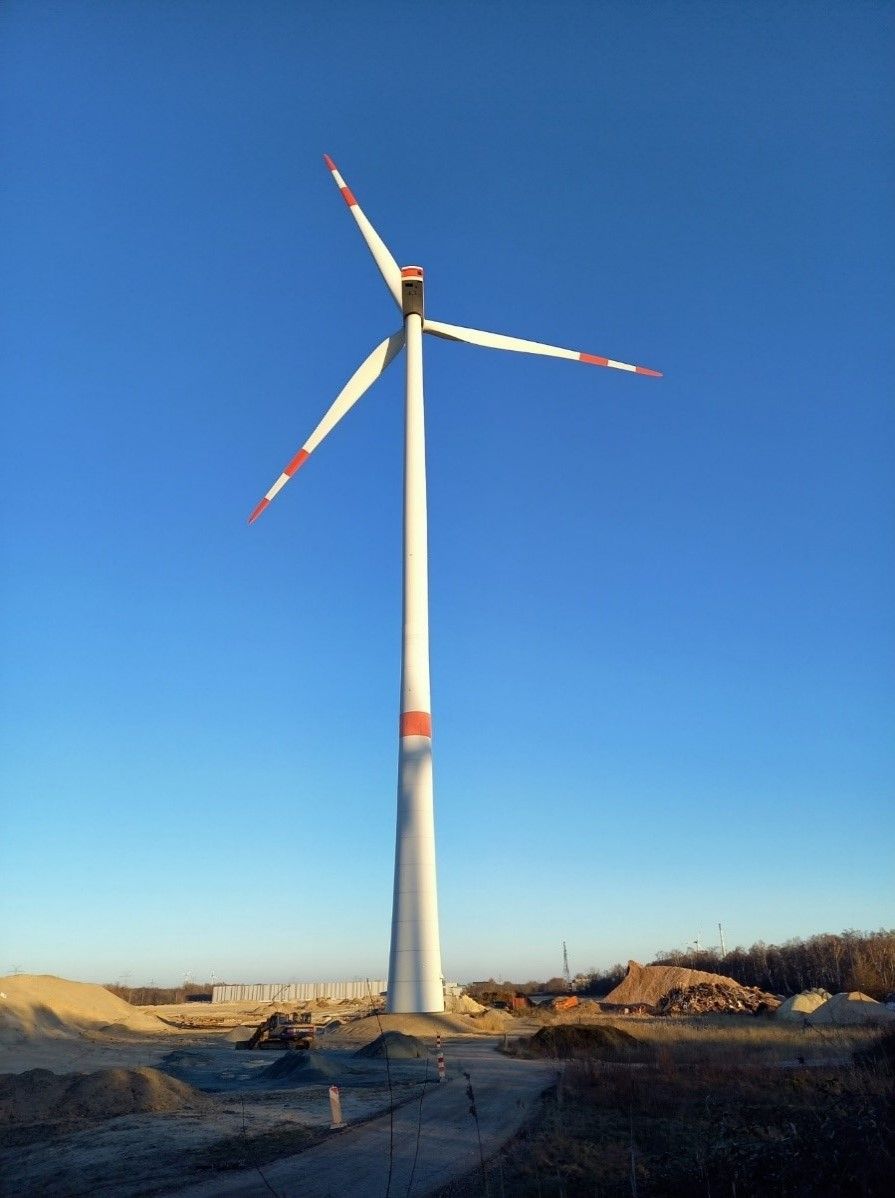
Publications
-
2024: Identification uncertainties of bending modes of an offshore wind turbine for vibration-based monitoring
Jonscher, C.; Möller, S.; Liesecke; L.; Schuster, D.; Hofmeister, B.; Grießmann, T.; Rolfes, R. (2024) Identification uncertainties of beding models of an offshore wind turbine for vibraion-based monitoring, Structural Control and Health Monitoring
-
2024: Heteroscedastic Gaussian processes for data normalisation in probablistic novelty detection of a wind turbine
Jonscher, C.; Möller, S.; Liesecke, L.; Hofmeister, B.; Grießmann, T.; Rolfes, R. (2024): Heteroscedastic Gaussian processes for data normalisation in probablistic novelty detection of a wind turbine, Engineering Structures 305, 117786
-
2024: Damage localisation using disparate damage states via domain adaption
Wickramarachchi, C.; Gardner, P.; Pool, J.; Hübler, C.; Jonscher, C.; Rolfes, R. (2024): Damage localisation using disparate damage states via domain adaption, Data-Centric Engineering 5, e3
-
2023: Investigations towards physics-informed gaussian process regression for the estimation of modal parameters of a lattice tower under environmental conditions. Experimental vibration analysis for civil engineering structures
Möller, S., Jonscher, C., Grießmann, T., Rolfes, R. (2023)
Investigations Towards Physics-Informed Gaussian Process Regression for the Estimation of Modal Parameters of a Lattice Tower Under Environmental Conditions. Experimental Vibration Analysis for Civil Engineering Structures
EVACES 2023. Lecture Notes in Civil Engineering 433. Springer, 401-410, 2023https://doi.org/10.1007/978-3-031-39117-0_41
-
2023: Validation of an FE model updating procedure for damage assessment using a modular laboratory experiment with a reversible damage mechanism
Wolniak, Marlene; Hofmeister, Benedikt; Jonscher, Clemens; Fankhänel, Matthias; Loose, Ansgar; Hübler, Clemens; Rolfes, Raimund (2023)
Validation of an FE model updating procedure for damage assessment using a modular laboratory experiment with a reversible damage mechanism
Journal of Civil Structural Health Monitoring -
2022: A new open‐database benchmark structure for vibration‐based Structural Health Monitoring
Wernitz, Stefan; Hofmeister, Benedikt; Jonscher, Clemens; Grießmann, Tanja; Rolfes, Raimund (2022)
A new open‐database benchmark structure for vibration‐based Structural Health Monitoring
Structural Control and Health Monitoring, 29 -
2022: On noise covariance estimation for Kalman filter-based damage localization
Wernitz, Stefan; Chatzi, Eleni; Hofmeister, Benedikt; Wolniak, Marlene; Shen, Wanzhou; Rolfes, Raimund (2022)
On noise covariance estimation for Kalman filter-based damage localization
Mechanical Systems and Signal Processing, 170 -
2022: Validierung von daten- und modellbasierten Methoden zur Schadenslokalisierung
Hübler, C., Hofmeister, B., Wernitz, S., & Rolfes, R. (2022)
Validierung von daten- und modellbasierten Methoden zur Schadenslokalisierung
Bautechnik, 6https://onlinelibrary.wiley.com/doi/abs/10.1002/bate.202200015
-
2021: Dataset: LUMO - Leibniz Universtity Test Structure for Monitoring
Stefan Wernitz, Benedikt Hofmeister, Clemens Jonscher, Tanja Grießmann, Raimund Rolfes (2021)
Dataset: LUMO - Leibniz Universtity Test Structure for Monitoring.
Subproject Management
30167 Hannover
Staff
30167 Hannover
30167 Hannover